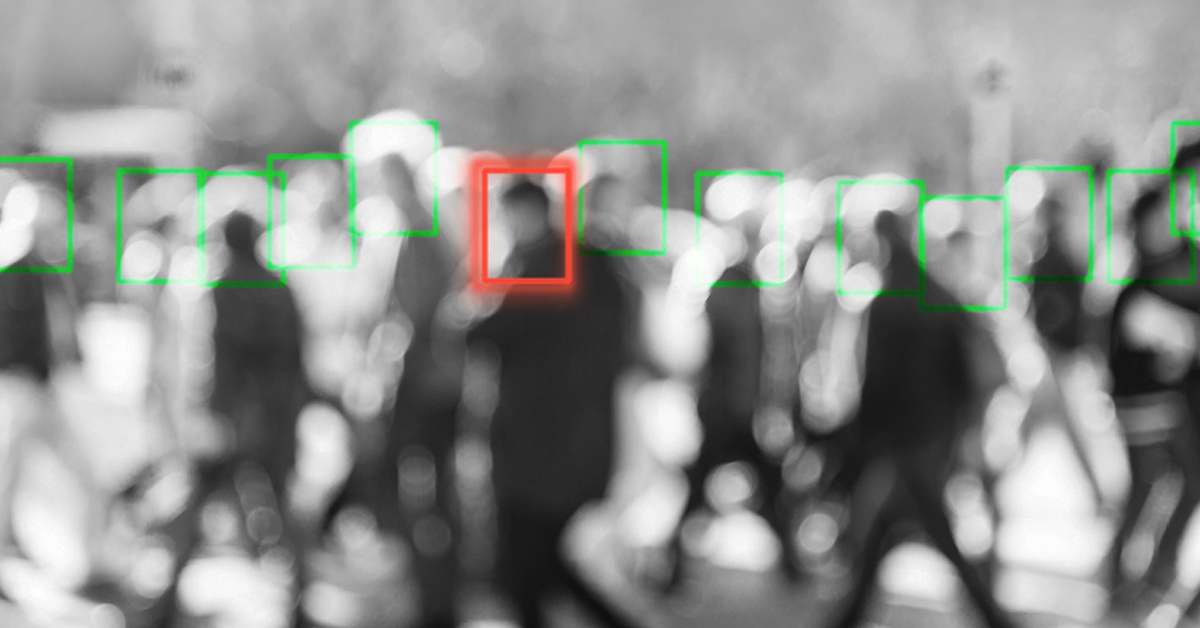
Each year, the National Institute of Standards and Technology (NIST) runs a Face Recognition Vendor Test (aka FRVT) designed to provide independent evaluations of commercially available and prototype face recognition technologies.
Founded in 1901, NIST is a non-regulatory federal agency within the U.S. Department of Commerce. NIST’s mission is to promote U.S. innovation and industrial competitiveness by advancing measurement science, standards, and technology in ways that enhance economic security and improve our quality of life.
How is the facial recognition testing done?
The FRVT measures the performance of automated face recognition technologies applied to a wide range of civil, law enforcement and homeland security applications including verification of visa images, de-duplication of passports, and recognition across photo journalism images. FRVT now has roughly 200 face recognition algorithms and tests against at least six collections of photographs with multiple sets of more than 8 million people. The best algorithms for 1:1 verification give false non-match rates of 0.0003 at false-match rates of 0.0001 on high-quality visa images.
5 Limitations of FRVT for Video Surveillance Use Cases
The good news with NIST is that we have an independent body that is testing third-party software – a la Consumer Reports or JD Power – and providing industry performance benchmarks. Unfortunately, many enterprises assume these tests are also applicable to video surveillance software – but they are not.
Make no mistake, FRVT serves its purpose. The testing is very relevant for a wide variety of use cases from access control to border control where the subject is looking directly at a camera and the image of that person is compared to a known watchlist or list of authorized employees. In these use cases, the image of the subject and the images on the watchlist are generally of high quality.
However, here are five reasons why enterprises should not use FRVT for comparing video surveillance solutions using facial recognition.
1. FRVT does not evaluate facial recognition solutions with real-time surveillance videos
The FRVT testing starts with a single input – a static photo image of a face, whether that be a mugshot, or a visa (photographic images are collected in association with a visa application) – and “wild” photos (pictures of an individual taken in common, everyday settings).
Software, such as Oosto’s OnWatch solution, work with video streams to identify specific individuals – usually as they’re entering a facility – to determine if they are on a watchlist. The AI and neural networks behind video-based facial recognition are fundamentally different from basic 1:1 and 1:n image recognition algorithms used by NIST.
2. FRVT does not address real-world conditions for video surveillance
Oosto’s algorithms are trained on real-world footage, and perform exceptionally well in adverse conditions such as low-lighting, poor angles, occlusions (like masks), extreme poses, and on very diverse datasets. In contrast, many of the vendors participating in NIST may perform well in controlled laboratory conditions, but do not perform as quickly or accurately with “in the wild” scenarios.
When I use the term “in the wild,” this is different from NIST’s definition of “wild” where people are not necessarily posing or cooperating for a photo. NIST’s wild images may be blurry, or of low quality, but the images are still relatively clear, the camera is generally at face level, and the lighting is good. In the real world, perhaps within a casino or stadium, subjects are far less cooperative and do not look directly at the camera. In these real-world scenarios, we often encounter extreme poses (given the camera location), poor image quality, low lighting, or occlusions such as hats, masks, or other coverings. This is a real test for facial recognition software.
In the image above, notice how blurry the video images are and the degree of occlusion for many faces. This is a radically more difficult problem than matching a still image of a person directly facing the camera in a well-lit environment.
3. FRVT does not evaluate facial recognition technologies designed to spot individuals within crowds
To be fair, NIST FRVT testing was never designed to evaluate technologies in crowded environments where there are streams of people entering a building at the same time. FRVT was designed for border control or (self-service) identity verification use cases. Unfortunately, many enterprises evaluating facial recognition solutions do not understand or appreciate this nuance until they have deployed a facial recognition solution and experience a high number of false positives and false negatives because the software, and its underlying algorithms, were not designed to identify individuals in crowds where there will naturally be higher degrees of occlusion and blurriness.
4. FRVT does not consider the quality of the video cameras
NIST FRVT testing makes certain assumptions about the quality of the cameras in place which are capturing images of subjects. Again, this makes sense when you consider the border control use cases in which FRVT was designed for. But, when you change the context to watchlist alerting – identifying bad actors or VIPs – in real time, then camera quality becomes very important. The performance of a face recognition system depends on the quality of both test and reference images participating in the face comparison process. Through AI and deep learning models, smart facial recognition cameras show dramatically improved accuracy and performance despite everyday challenges such as long distances, low-resolution videos or situations when the faces are not directed towards the camera. Enterprises that use high-resolution, surveillance cameras are able to recognize specific individuals from a variety of camera angles, even in dim light, and can have a dramatic impact on facial recognition accuracy.
5. FRVT mounts cameras at face-level
Facial recognition algorithms tend to have good accuracy on NIST’s verification tasks, because the subject usually knows they are being scanned and can position themselves to give their cameras a clear view of their face. In more controlled settings (e.g., border control or access control scenarios), the images used for facial recognition are often “well lit,” meaning the illumination is full, with no shadows or over illumination.
But this is generally not the case when it comes to video surveillance in the wild, such as with networked CCTV cameras. Think about a stadium trying to identify a known hooligan or a casino trying to identify advantage players; the CCTV cameras are often mounted high on a wall or ceiling. As an image containing faces degrades from “headshot quality” to “grainy low light,” facial recognition algorithms and neural networks have to work harder.
As NIST notes: “Poor quality photographs undermine recognition, either because the imaging system is poor or because the subject mis-presents to the camera” (head orientation, facial expression, occlusion, etc.).
This is not an indictment
This post is not intended to criticize NIST. It was created to help enterprise buyers better understand the purpose and limitations of NIST’s FRVT testing and reporting. If your organization is evaluating solutions where a static photo of a person is presented and you need to compare that image against a database of photographs, then FRVT is a good means of comparing one vendor against another (with some of the stated caveats understood).
But, for most video surveillance use cases where video analytics are being leveraged for real-time facial recognition detection, FRVT is wholly inadequate and inappropriate as a benchmark.
We encourage NIST to create a new testing methodology to better compare video surveillance solutions with facial recognition that factor in real-world conditions such as poor lighting, occlusion, and challenging camera angles and even camera quality. This would obviously help commercial buyers understand the true performance of these video surveillance solutions instead of relying on FRVT tests that do not mimic the real-world.