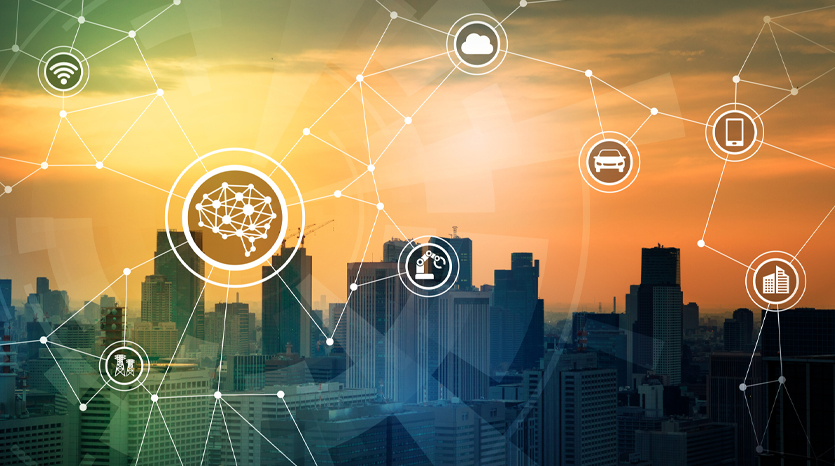
The edge is exploding.
According to the “2021 IoT and Edge Commercial Adoption Survey” from the Eclipse Foundation, 54% of organizations are either using or planning to use edge computing technologies within 12 months, with another 30% planning to evaluate edge deployments within the next two years. Additionally, 30% expect to spend between $100,000 to $1 million on those initiatives, and 16% plan to spend more than $1 million.
But why now?
There are a number of reasons why edge computing is starting to gain real traction in the market.
-
- Too much data. Moving all this critical data to the cloud and utilizing it to create meaningful insights is increasingly becoming a challenge for enterprises. It’s especially important for real-time insights.
- Too much latency. The edge reduces latency, decreases the need for large amounts of expensive network bandwidth to the cloud, and enables mission-critical operations to continue even when the network to the cloud is down.
- Too little security. Edge computing can provide enhanced security and more privacy protections because it keeps data close to the edge and thus out of centralized servers. Because the data is not being transferred to the cloud, there’s less opportunity to steal the information in transit. The edge can dramatically reduce the vulnerable attack surface of many applications.
- Too little connectivity. Besides latency, edge computing is preferred over cloud computing in remote locations, where there is limited or no connectivity to a centralized location. These locations require local storage, similar to a mini data center, with edge computing providing the perfect solution for it.
A Perfect Pairing: The Edge and Video Analytics
Back in 2014, when edge computing was just being conceived, Microsoft searched for the killer app that could exploit all of the edge’s benefits. They ultimately settled on video analytics. Here’s an excerpt from Victor Bahl’s blog post titled, “10 years is an eternity in the tech world, but we are just getting started.” Mr. Bahl is Microsoft’s Technical Fellow & Chief Technology Officer, Azure for Operators.
According to some reports, there were tens of millions of cameras in major cities. So how were they being used? I imagined every time there was an incident, authorities would have to go to the stored video stream to find the recording that had captured the event and then analyze it. Instead, why not have computers analyze these streams in real-time and generate a workflow whenever an anomaly was detected? Computers are good at such things.
For this to work, we would need cloud-like compute resources, and they would have to be close to the cameras because the system would have to analyze large quantities of data quickly. Furthermore, the cost of streaming every video stream to the cloud could be prohibitive, plus add to it the expense of renting GPUs in the cloud to process each of these streams. This was the perfect scenario — the killer app for edge computing — and it would solve a compelling real-world, large-scale problem.
The Edge and Real-Time Video Surveillance
It was only a matter of time before real-time video surveillance systems would harness the power of the edge to transfer compute workloads from expensive on-premise servers to the edge. After all, significant processing power is required to analyze every frame of a video feed and perform a variety of instant computations to determine if the person entering a building is an authorized employee or on a watchlist (e.g., a VIP or security threat).
Within the video surveillance space, two flavors of edge computing have emerged:
- Pure Edge Computing: Video processing happens within the camera itself rather than on the back end. With pure edge computing, the neural networks are optimized on the actual chips (via an SDK), enabling low-power, high-resolution IP security cameras with features such as facial recognition, person detection, analytics, and more.
- Near-Edge Appliances: With near-edge appliances, the video processing happens on an appliance (which is connected to the camera streams). The neural networks must be optimized to support low-power devices while still offering superior performance and recognition accuracy. Historically, expensive and energy-intensive GPU processing servers were required to address the heavy compute needs for real-time video analytics.
Over time, pure edge computing via AI CCTV cameras shows considerable promise, but adoption will presumably be slow and steady. When neural networks can operate at the chip level, AI CCTV cameras send their video streams to a recorder and are processed via an AI layer to make sense of the raw video. This enables them to deliver advanced analytical functions like vehicle detection, face detection, person detection, people counting, traffic counting, and license plate recognition.
Uneven Adoption for AI Cameras
There’s no doubt that the AI camera market will grow and grow fast. The global AI CCTV market size was valued at $14.8 billion in 2020 and is projected to reach $55.2 billion by 2030, registering a CAGR of 14.9% from 2021 to 2030 (source: Allied Market Research, August 2021). But adoption in the near term for AI cameras is probably more limited to greenfield projects.
A greenfield opportunity refers to a project for a totally new environment and requires development from a clean slate – that is, you’re installing a video surveillance system from scratch. By comparison, a brownfield project refers to the development and deployment of a video surveillance system in the presence of an existing or legacy system (i.e., your cameras are already in place).
There are often forces at work that can slow the adoption of AI CCTV cameras. According to Sarah Berz, AI Product Manager at Jalgos: “The costs involved in overhauling legacy camera systems, converting videos into higher quality, or waiting until the current camera network is ready to be replaced in order to integrate AI is expensive, time-consuming, and often unfeasible.”
This is where near-edge compute appliances fit in. They enable organizations to reap the benefits of real-time video analytics without ripping and replacing their existing camera infrastructure. Near-edge compute appliances can solve a number of real-world benefits for real-time video analytics, including:
- TCO (Total Cost of Ownership) Savings: By pushing more analytic capabilities closer to where data is collected (i.e., the cameras), enterprises can achieve greater responsiveness, efficiency, and TCO savings with a small, low-power, near-edge appliance.
- Scalability: When new video streams are added, organizations can simply add more near-edge compute appliances without taking the entire system down, improving overall uptime.
- IT Simplicity: These compact appliances do not take up space in a rack, consume much power, or place a burden on cooling systems. Having a “ruggedized” device without fans or moving parts allows the appliances to obtain a much longer operating life, leading to fewer failures and an overall reduction in cost, and channel redundancy.
- Enterprise-Class Failover: If an appliance fails, the near-edge device can failover to a secondary, low-cost appliance that can easily absorb the payload of the failed appliance.
- More algorithms mean more compute power: Even if you invest in AI CCTV cameras, you can still benefit from near-edge compute appliances. The fact is that AI cameras can only run a limited number of algorithms. Your organization may still need a near-edge device to run additional AI algorithms such as body or object recognition — beyond what is being run on the camera itself. The near-edge gives you the needed horsepower to run additional and more complex real-time video analytics/algorithms.
- Convert your dumb cameras into smart ones: Near-edge appliances work with your existing camera infrastructure and can effectively transform dumb cameras into smart cameras by analyzing the video frames in real-time and generating actionable intelligence (e.g., recognizing a VIP as he/she walks through the front doors).
- Data Security: Traditional servers used for video processing are often open-boxes, and unused services and open ports can introduce security vulnerabilities. Near-edge appliances are purpose-built closed-boxes which translates to a much smaller surface area for attack.
Against this backdrop, Oosto is announcing the arrival of the Oosto Vision AI Appliance, a revolutionary near-edge device that delivers the power and security of Vision AI in a palm-sized device.
The Vision AI Appliance is based on the NVIDIA® Jetson Xavier™ NX system on module and equipped with Oosto’s state-of-the-art neural network models for video analytics that are optimized to support low-power devices. Significantly, this was achieved without compromising on superior performance, security, or recognition accuracy which historically required compute-intensive GPU processing servers.
If I’ve piqued your interest, check out www.oosto.com/visionai-appliance to learn more, watch a quick video, and even explore our TCO calculator which allows you to estimate the annual cost savings of the Vision AI Appliance (compared to traditional hardware-centric configurations used for real-time video analytics).